Week 9 [Mar 18]
[W9.1] Integration Approaches
Can explain how integration approaches vary based on timing and frequency
In terms of timing and frequency, there are two general approaches to integration: late and one-time, early and frequent.
Late and one-time: wait till all components are completed and integrate all finished components near the end of the project.
This approach is not recommended because integration often causes many component incompatibilities (due to previous miscommunications and misunderstandings) to surface which can lead to delivery delays i.e. Late integration → incompatibilities found → major rework required → cannot meet the delivery date.
Early and frequent: integrate early and evolve each part in parallel, in small steps, re-integrating frequently.
A
Here is an animation that compares the two approaches:
Can explain how integration approaches vary based on amount merged at a time
Big-bang integration: integrate all components at the same time.
Big-bang is not recommended because it will uncover too many problems at the same time which could make debugging and bug-fixing more complex than when problems are uncovered incrementally.
Incremental integration: integrate few components at a time. This approach is better than the big-bang integration because it surfaces integration problems in a more manageable way.
Here is an animation that compares the two approaches:
Give two arguments in support and two arguments against the following statement.
Because there is no external client, it is OK to use big bang integration for a school project.
Arguments for:
- It is relatively simple; even big-bang can succeed.
- Project duration is short; there is not enough time to integrate in steps.
- The system is non-critical, non-production (demo only); the cost of integration issues is relatively small.
Arguments against:
- Inexperienced developers; big-bang more likely to fail
- Too many problems may be discovered too late. Submission deadline (fixed) can be missed.
- Team members have not worked together before; increases the probability of integration problems.
Can explain how integration approaches vary based on the order of integration
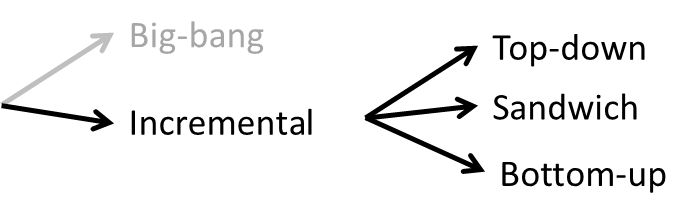
Based on the order in which components are integrated, incremental integration can be done in three ways.
Top-down integration: higher-level components are integrated before bringing in the lower-level components. One advantage of this approach is that higher-level problems can be discovered early. One disadvantage is that this requires the use of
Stub: A stub has the same interface as the component it replaces, but its implementation is so simple that it is unlikely to have any bugs. It mimics the responses of the component, but only for the a limited set of predetermined inputs. That is, it does not know how to respond to any other inputs. Typically, these mimicked responses are hard-coded in the stub rather than computed or retrieved from elsewhere, e.g. from a database.
Bottom-up integration: the reverse of top-down integration. Note that when integrating lower level components,
Sandwich integration: a mix of the top-down and the bottom-up approaches. The idea is to do both top-down and bottom-up so as to 'meet' in the middle.
Here is an animation that compares the three approaches:
Suggest an integration strategy for the system represented by following diagram. You need not follow a strict top-down, bottom-up, sandwich, or big bang approach. Dashed arrows represent dependencies between classes.
Also take into account the following facts in your test strategy.
HospitalUI
will be developed early, so as to get customer feedback early.HospitalFacade
shields the UI from complexities of the application layer. It simply redirects the method calls received to the appropriate classes belowIO_Helper
is to be reused from an earlier project, with minor modifications- Development of
OutPatient
component has been outsourced, and the delivery is not expected until the 2nd half of the project.
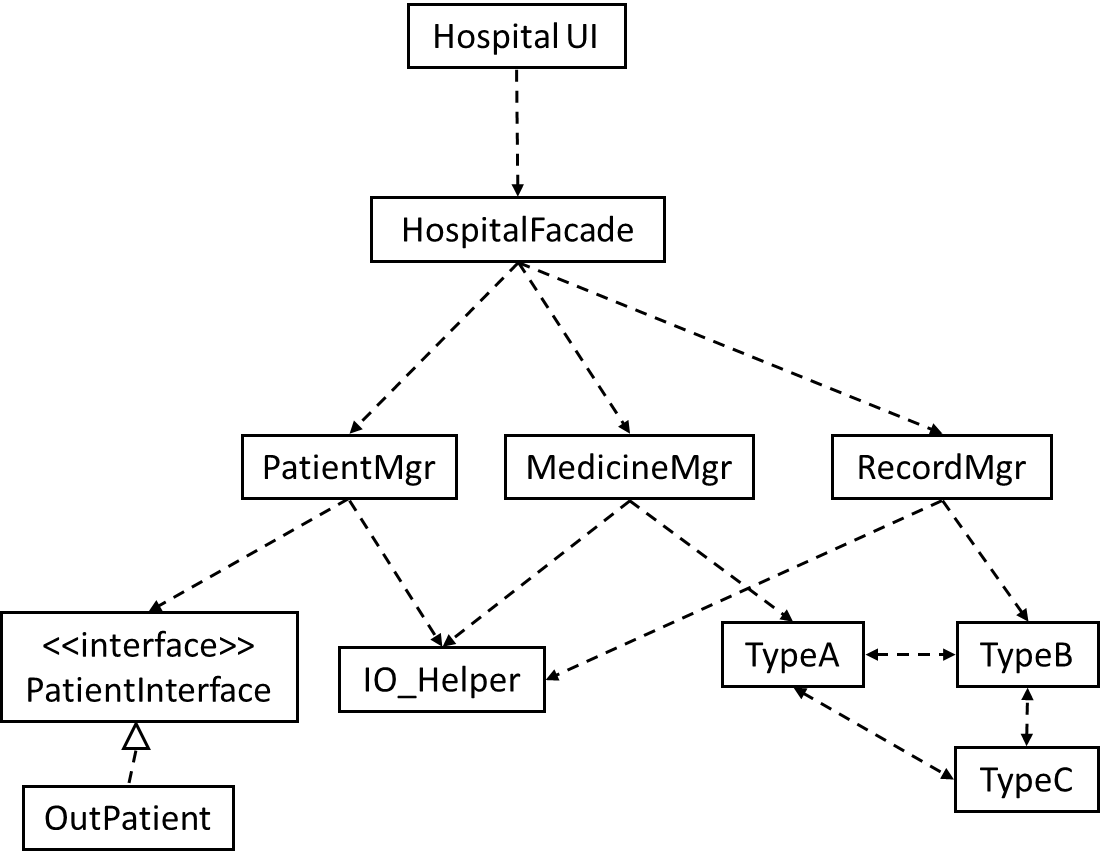
There can be many acceptable answers to this question. But any good strategy should consider at least some of the below.
- Because
HospitalUI
will be developed early, it’s OK to integrate it early, using stubs, rather than wait for the rest of the system to finish. (i.e. a top-down integration is suitable forHospitalUI
) - Because
HospitalFacade
is unlikely to have a lot of business logic, it may not be worth to write stubs to test it (i.e. a bottom-up integration is better forHospitalFacade
). - Because
IO_Helper
is to be reused from an earlier project, we can finish it early. This is especially suitable since there are many classes that use it. ThereforeIO_Helper
can be integrated with the dependent classes in bottom-up fashion. - Because
OutPatient
class may be delayed, we may have to integratePatientMgr
using a stub. TypeA
,TypeB
, andTypeC
seem to be tightly coupled. It may be a good idea to test them together.
Given below is one possible integration test strategy. Relative positioning also indicates a rough timeline.
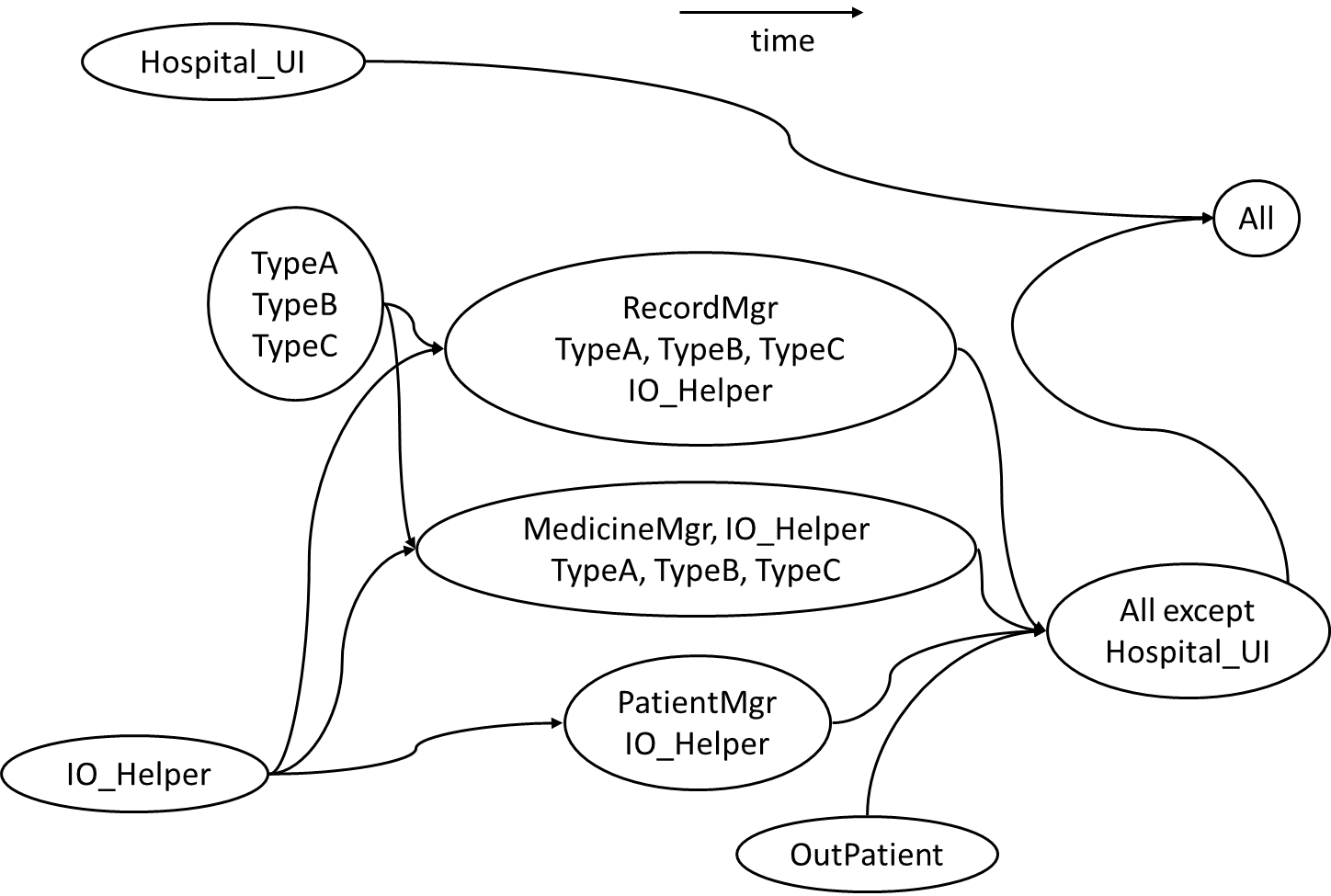
Consider the architecture given below. Describe the order in which components will be integrated with one another if the following integration strategies were adopted.
a) top-down b) bottom-up c) sandwich
Note that dashed arrows show dependencies (e.g. A depend on B, C, D and therefore, higher-level than B, C and D).
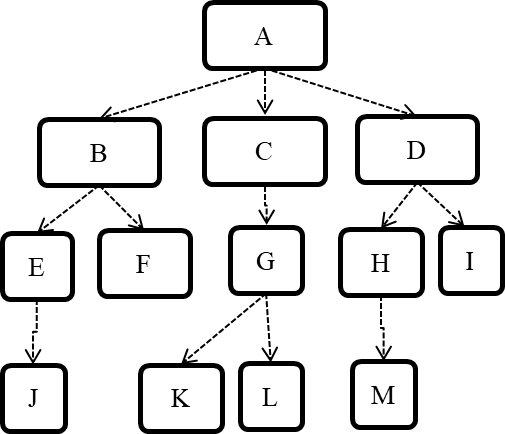
a) Diagram:
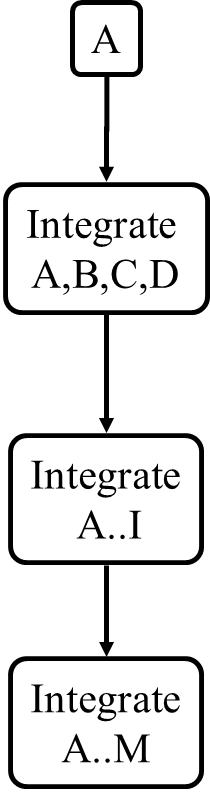
b) Diagram:
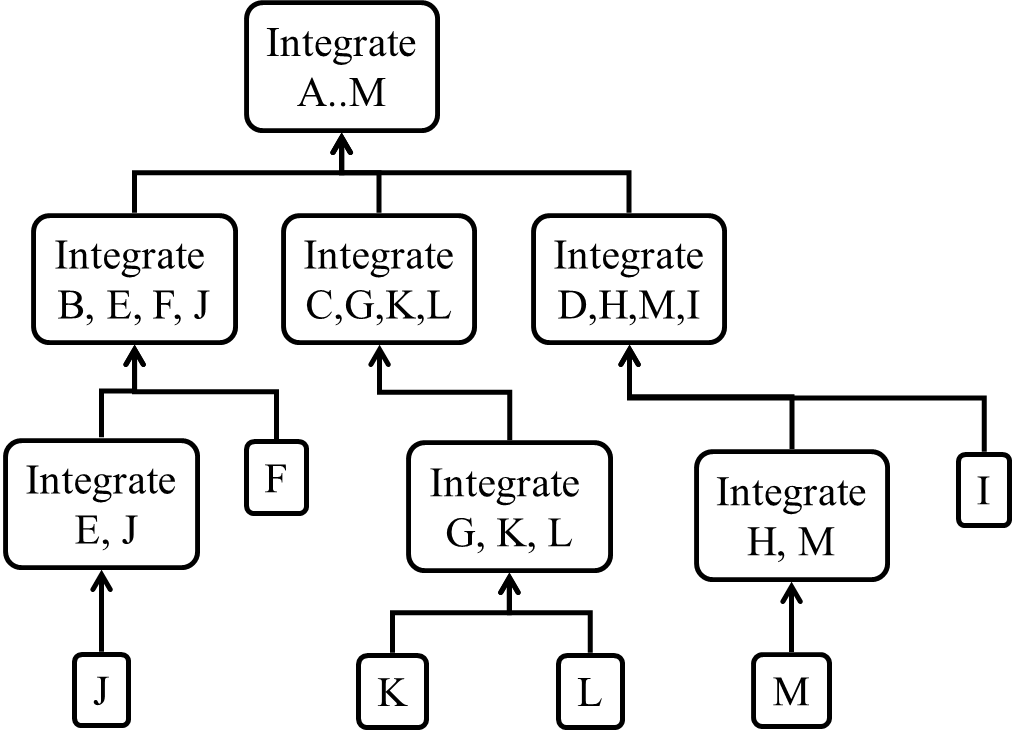
c) Diagram:
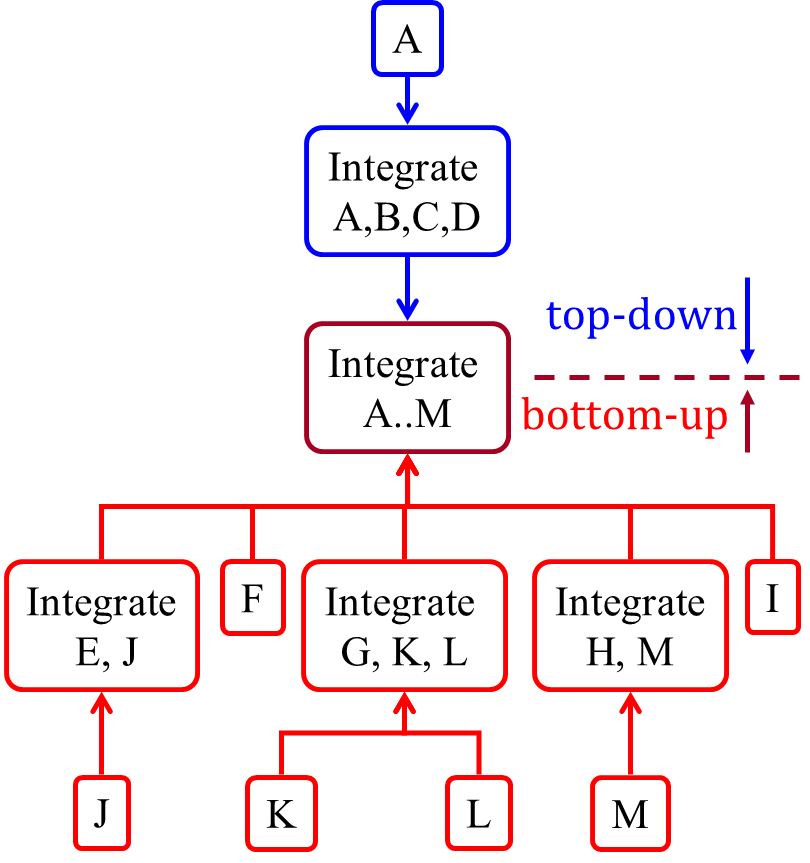
[W9.2] Types of Testing
Unit Testing
Can explain unit testing
Unit testing : testing individual units (methods, classes, subsystems, ...) to ensure each piece works correctly.
In OOP code, it is common to write one or more unit tests for each public method of a class.
Here are the code skeletons for a Foo
class containing two methods and a FooTest
class that contains unit tests for those two methods.
class Foo{
String read(){
//...
}
void write(String input){
//...
}
}
class FooTest{
@Test
void read(){
//a unit test for Foo#read() method
}
@Test
void write_emptyInput_exceptionThrown(){
//a unit tests for Foo#write(String) method
}
@Test
void write_normalInput_writtenCorrectly(){
//another unit tests for Foo#write(String) method
}
}
import unittest
class Foo:
def read(self):
# ...
def write(self, input):
# ...
class FooTest(unittest.TestCase):
def test_read(sefl):
# a unit test for read() method
def test_write_emptyIntput_ignored(self):
# a unit tests for write(string) method
def test_write_normalInput_writtenCorrectly(self):
# another unit tests for write(string) method
Side readings:
- [Web article] The three pillars of unit testing - A short article about what makes a good unit test.
- Learning from Apple’s #gotofail Security Bug - How unit testing (and other good coding practices) could have prevented a major security bug.
Can use stubs to isolate an SUT from its dependencies
A proper unit test requires the unit to be tested in isolation so that bugs in the
If a Logic
class depends on a Storage
class, unit testing the Logic
class requires isolating the Logic
class from the Storage
class.
Stubs can isolate the
Stub: A stub has the same interface as the component it replaces, but its implementation is so simple that it is unlikely to have any bugs. It mimics the responses of the component, but only for the a limited set of predetermined inputs. That is, it does not know how to respond to any other inputs. Typically, these mimicked responses are hard-coded in the stub rather than computed or retrieved from elsewhere, e.g. from a database.
Consider the code below:
class Logic {
Storage s;
Logic(Storage s) {
this.s = s;
}
String getName(int index) {
return "Name: " + s.getName(index);
}
}
interface Storage {
String getName(int index);
}
class DatabaseStorage implements Storage {
@Override
public String getName(int index) {
return readValueFromDatabase(index);
}
private String readValueFromDatabase(int index) {
// retrieve name from the database
}
}
Normally, you would use the Logic
class as follows (not how the Logic
object depends on a DatabaseStorage
object to perform the getName()
operation):
Logic logic = new Logic(new DatabaseStorage());
String name = logic.getName(23);
You can test it like this:
@Test
void getName() {
Logic logic = new Logic(new DatabaseStorage());
assertEquals("Name: John", logic.getName(5));
}
However, this logic
object being tested is making use of a DataBaseStorage
object which means a bug in the DatabaseStorage
class can affect the test. Therefore, this test is not testing Logic
in isolation from its dependencies and hence it is not a pure unit test.
Here is a stub class you can use in place of DatabaseStorage
:
class StorageStub implements Storage {
@Override
public String getName(int index) {
if(index == 5) {
return "Adam";
} else {
throw new UnsupportedOperationException();
}
}
}
Note how the stub has the same interface as the real dependency, is so simple that it is unlikely to contain bugs, and is pre-configured to respond with a hard-coded response, presumably, the correct response DatabaseStorage
is expected to return for the given test input.
Here is how you can use the stub to write a unit test. This test is not affected by any bugs in the DatabaseStorage
class and hence is a pure unit test.
@Test
void getName() {
Logic logic = new Logic(new StorageStub());
assertEquals("Name: Adam", logic.getName(5));
}
In addition to Stubs, there are other type of replacements you can use during testing. E.g. Mocks, Fakes, Dummies, Spies.
- Mocks Aren't Stubs by Martin Fowler -- An in-depth article about how Stubs differ from other types of test helpers.
Stubs help us to test a component in isolation from its dependencies.
True
Can explain dependency injection
Dependency injection is the process of 'injecting' objects to replace current dependencies with a different object. This is often used to inject
Quality Assurance → Testing → Unit Testing →
A proper unit test requires the unit to be tested in isolation so that bugs in the
If a Logic
class depends on a Storage
class, unit testing the Logic
class requires isolating the Logic
class from the Storage
class.
Stubs can isolate the
Stub: A stub has the same interface as the component it replaces, but its implementation is so simple that it is unlikely to have any bugs. It mimics the responses of the component, but only for the a limited set of predetermined inputs. That is, it does not know how to respond to any other inputs. Typically, these mimicked responses are hard-coded in the stub rather than computed or retrieved from elsewhere, e.g. from a database.
Consider the code below:
class Logic {
Storage s;
Logic(Storage s) {
this.s = s;
}
String getName(int index) {
return "Name: " + s.getName(index);
}
}
interface Storage {
String getName(int index);
}
class DatabaseStorage implements Storage {
@Override
public String getName(int index) {
return readValueFromDatabase(index);
}
private String readValueFromDatabase(int index) {
// retrieve name from the database
}
}
Normally, you would use the Logic
class as follows (not how the Logic
object depends on a DatabaseStorage
object to perform the getName()
operation):
Logic logic = new Logic(new DatabaseStorage());
String name = logic.getName(23);
You can test it like this:
@Test
void getName() {
Logic logic = new Logic(new DatabaseStorage());
assertEquals("Name: John", logic.getName(5));
}
However, this logic
object being tested is making use of a DataBaseStorage
object which means a bug in the DatabaseStorage
class can affect the test. Therefore, this test is not testing Logic
in isolation from its dependencies and hence it is not a pure unit test.
Here is a stub class you can use in place of DatabaseStorage
:
class StorageStub implements Storage {
@Override
public String getName(int index) {
if(index == 5) {
return "Adam";
} else {
throw new UnsupportedOperationException();
}
}
}
Note how the stub has the same interface as the real dependency, is so simple that it is unlikely to contain bugs, and is pre-configured to respond with a hard-coded response, presumably, the correct response DatabaseStorage
is expected to return for the given test input.
Here is how you can use the stub to write a unit test. This test is not affected by any bugs in the DatabaseStorage
class and hence is a pure unit test.
@Test
void getName() {
Logic logic = new Logic(new StorageStub());
assertEquals("Name: Adam", logic.getName(5));
}
In addition to Stubs, there are other type of replacements you can use during testing. E.g. Mocks, Fakes, Dummies, Spies.
- Mocks Aren't Stubs by Martin Fowler -- An in-depth article about how Stubs differ from other types of test helpers.
Stubs help us to test a component in isolation from its dependencies.
True
A Foo
object normally depends on a Bar
object, but we can inject a BarStub
object so that the Foo
object no longer depends on a Bar
object. Now we can test the Foo
object in isolation from the Bar
object.
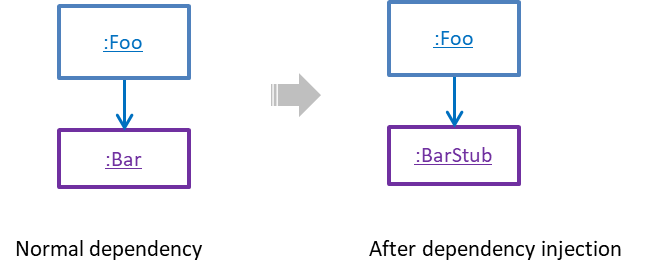
Can use dependency injection
Polymorphism can be used to implement dependency injection, as can be seen in the example given in
Quality Assurance → Testing → Unit Testing →
A proper unit test requires the unit to be tested in isolation so that bugs in the
If a Logic
class depends on a Storage
class, unit testing the Logic
class requires isolating the Logic
class from the Storage
class.
Stubs can isolate the
Stub: A stub has the same interface as the component it replaces, but its implementation is so simple that it is unlikely to have any bugs. It mimics the responses of the component, but only for the a limited set of predetermined inputs. That is, it does not know how to respond to any other inputs. Typically, these mimicked responses are hard-coded in the stub rather than computed or retrieved from elsewhere, e.g. from a database.
Consider the code below:
class Logic {
Storage s;
Logic(Storage s) {
this.s = s;
}
String getName(int index) {
return "Name: " + s.getName(index);
}
}
interface Storage {
String getName(int index);
}
class DatabaseStorage implements Storage {
@Override
public String getName(int index) {
return readValueFromDatabase(index);
}
private String readValueFromDatabase(int index) {
// retrieve name from the database
}
}
Normally, you would use the Logic
class as follows (not how the Logic
object depends on a DatabaseStorage
object to perform the getName()
operation):
Logic logic = new Logic(new DatabaseStorage());
String name = logic.getName(23);
You can test it like this:
@Test
void getName() {
Logic logic = new Logic(new DatabaseStorage());
assertEquals("Name: John", logic.getName(5));
}
However, this logic
object being tested is making use of a DataBaseStorage
object which means a bug in the DatabaseStorage
class can affect the test. Therefore, this test is not testing Logic
in isolation from its dependencies and hence it is not a pure unit test.
Here is a stub class you can use in place of DatabaseStorage
:
class StorageStub implements Storage {
@Override
public String getName(int index) {
if(index == 5) {
return "Adam";
} else {
throw new UnsupportedOperationException();
}
}
}
Note how the stub has the same interface as the real dependency, is so simple that it is unlikely to contain bugs, and is pre-configured to respond with a hard-coded response, presumably, the correct response DatabaseStorage
is expected to return for the given test input.
Here is how you can use the stub to write a unit test. This test is not affected by any bugs in the DatabaseStorage
class and hence is a pure unit test.
@Test
void getName() {
Logic logic = new Logic(new StorageStub());
assertEquals("Name: Adam", logic.getName(5));
}
In addition to Stubs, there are other type of replacements you can use during testing. E.g. Mocks, Fakes, Dummies, Spies.
- Mocks Aren't Stubs by Martin Fowler -- An in-depth article about how Stubs differ from other types of test helpers.
Stubs help us to test a component in isolation from its dependencies.
True
Here is another example of using polymorphism to implement dependency injection:
Suppose we want to unit test the Payroll#totalSalary()
given below. The method depends on the SalaryManager
object to calculate the return value. Note how the setSalaryManager(SalaryManager)
can be used to inject a SalaryManager
object to replace the current SalaryManager
object.
class Payroll {
private SalaryManager manager = new SalaryManager();
private String[] employees;
void setEmployees(String[] employees) {
this.employees = employees;
}
void setSalaryManager(SalaryManager sm) {
this. manager = sm;
}
double totalSalary() {
double total = 0;
for(int i = 0;i < employees.length; i++){
total += manager.getSalaryForEmployee(employees[i]);
}
return total;
}
}
class SalaryManager {
double getSalaryForEmployee(String empID){
//code to access employee’s salary history
//code to calculate total salary paid and return it
}
}
During testing, you can inject a SalaryManagerStub
object to replace the SalaryManager
object.
class PayrollTest {
public static void main(String[] args) {
//test setup
Payroll p = new Payroll();
p.setSalaryManager(new SalaryManagerStub()); //dependency injection
//test case 1
p.setEmployees(new String[]{"E001", "E002"});
assertEquals(2500.0, p.totalSalary());
//test case 2
p.setEmployees(new String[]{"E001"});
assertEquals(1000.0, p.totalSalary());
//more tests ...
}
}
class SalaryManagerStub extends SalaryManager {
/** Returns hard coded values used for testing */
double getSalaryForEmployee(String empID) {
if(empID.equals("E001")) {
return 1000.0;
} else if(empID.equals("E002")) {
return 1500.0;
} else {
throw new Error("unknown id");
}
}
}
Choose correct statement about dependency injection
- a. It is a technique for increasing dependencies
- b. It is useful for unit testing
- c. It can be done using polymorphism
- d. It can be used to substitute a component with a stub
(a)(b)(c)(d)
Explanation: It is a technique we can use to substitute an existing dependency with another, not increase dependencies. It is useful when you want to test a component in isolation but the SUT depends on other components. Using dependency injection, we can substitute those other components with test-friendly stubs. This is often done using polymorphism.
Integration Testing
Can explain integration testing
Integration testing : testing whether different parts of the software work together (i.e. integrates) as expected. Integration tests aim to discover bugs in the 'glue code' related to how components interact with each other. These bugs are often the result of misunderstanding of what the parts are supposed to do vs what the parts are actually doing.
Suppose a class Car
users classes Engine
and Wheel
. If the Car
class assumed a Wheel
can support 200 mph speed but the actual Wheel
can only support 150 mph, it is the integration test that is supposed to uncover this discrepancy.
Can use integration testing
Integration testing is not simply a repetition of the unit test cases but run using the actual dependencies (instead of the stubs used in unit testing). Instead, integration tests are additional test cases that focus on the interactions between the parts.
Suppose a class Car
uses classes Engine
and Wheel
. Here is how you would go about doing pure integration tests:
a) First, unit test Engine
and Wheel
.
b) Next, unit test Car
in isolation of Engine
and Wheel
, using stubs for Engine
and Wheel
.
c) After that, do an integration test for Car
using it together with the Engine
and Wheel
classes to ensure the Car
integrates properly with the Engine
and the Wheel
.
In practice, developers often use a hybrid of unit+integration tests to minimize the need for stubs.
Here's how a hybrid unit+integration approach could be applied to the same example used above:
(a) First, unit test Engine
and Wheel
.
(b) Next, unit test Car
in isolation of Engine
and Wheel
, using stubs for Engine
and Wheel
.
(c) After that, do an integration test for Car
using it together with the Engine
and Wheel
classes to ensure the Car
integrates properly with the Engine
and the Wheel
. This step should include test cases that are meant to test the unit Car
(i.e. test cases used in the step (b) of the example above) as well as test cases that are meant to test the integration of Car
with Wheel
and Engine
(i.e. pure integration test cases used of the step (c) in the example above).
💡 Note that you no longer need stubs for Engine
and Wheel
. The downside is that Car
is never tested in isolation of its dependencies. Given that its dependencies are already unit tested, the risk of bugs in Engine
and Wheel
affecting the testing of Car
can be considered minimal.
System Testing
Can explain system testing
System testing: take the whole system and test it against the system specification.
System testing is typically done by a testing team (also called a QA team).
System test cases are based on the specified external behavior of the system. Sometimes, system tests go beyond the bounds defined in the specification. This is useful when testing that the system fails 'gracefully' having pushed beyond its limits.
Suppose the SUT is a browser supposedly capable of handling web pages containing up to 5000 characters. Given below is a test case to test if the SUT fails gracefully if pushed beyond its limits.
Test case: load a web page that is too big
* Input: load a web page containing more than 5000 characters.
* Expected behavior: abort the loading of the page and show a meaningful error message.
This test case would fail if the browser attempted to load the large file anyway and crashed.
System testing includes testing against non-functional requirements too. Here are some examples.
- Performance testing – to ensure the system responds quickly.
- Load testing (also called stress testing or scalability testing) – to ensure the system can work under heavy load.
- Security testing – to test how secure the system is.
- Compatibility testing, interoperability testing – to check whether the system can work with other systems.
- Usability testing – to test how easy it is to use the system.
- Portability testing – to test whether the system works on different platforms.
Can explain automated GUI testing
If a software product has a GUI component, all product-level testing (i.e. the types of testing mentioned above) need to be done using the GUI. However, testing the GUI is much harder than testing the CLI (command line interface) or API, for the following reasons:
- Most GUIs can support a large number of different operations, many of which can be performed in any arbitrary order.
- GUI operations are more difficult to automate than API testing. Reliably automating GUI operations and automatically verifying whether the GUI behaves as expected is harder than calling an operation and comparing its return value with an expected value. Therefore, automated regression testing of GUIs is rather difficult.
- The appearance of a GUI (and sometimes even behavior) can be different across platforms and even environments. For example, a GUI can behave differently based on whether it is minimized or maximized, in focus or out of focus, and in a high resolution display or a low resolution display.
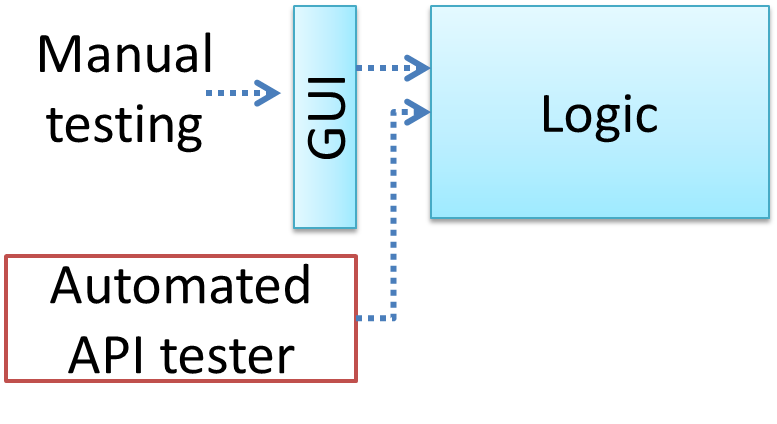
One approach to overcome the challenges of testing GUIs is to minimize logic aspects in the GUI. Then, bypass the GUI to test the rest of the system using automated API testing. While this still requires the GUI to be tested manually, the number of such manual test cases can be reduced as most of the system has been tested using automated API testing.
There are testing tools that can automate GUI testing.
Some tools used for automated GUI testing:
GUI testing is usually easier than API testing because it doesn’t require any extra coding.
False
Acceptance Testing
Can explain acceptance testing
Acceptance testing (aka User Acceptance Testing (UAT)): test the delivered system to ensure it meets the user requirements.
Acceptance tests give an assurance to the customer that the system does what it is intended to do. Acceptance test cases are often defined at the beginning of the project, usually based on the use case specification. Successful completion of UAT is often a prerequisite to the project sign-off.
Can explain the differences between system testing and acceptance testing
Acceptance testing comes after system testing. Similar to system testing, acceptance testing involves testing the whole system.
Some differences between system testing and acceptance testing:
System Testing | Acceptance Testing |
---|---|
Done against the system specification | Done against the requirements specification |
Done by testers of the project team | Done by a team that represents the customer |
Done on the development environment or a test bed | Done on the deployment site or on a close simulation of the deployment site |
Both negative and positive test cases | More focus on positive test cases |
Note: negative test cases: cases where the SUT is not expected to work normally e.g. incorrect inputs; positive test cases: cases where the SUT is expected to work normally
Requirement Specification vs System Specification
The requirement specification need not be the same as the system specification. Some example differences:
Requirements Specification | System Specification |
---|---|
limited to how the system behaves in normal working conditions | can also include details on how it will fail gracefully when pushed beyond limits, how to recover, etc. specification |
written in terms of problems that need to be solved (e.g. provide a method to locate an email quickly) | written in terms of how the system solve those problems (e.g. explain the email search feature) |
specifies the interface available for intended end-users | could contain additional APIs not available for end-users (for the use of developers/testers) |
However, in many cases one document serves as both a requirement specification and a system specification.
Passing system tests does not necessarily mean passing acceptance testing. Some examples:
- The system might work on the testbed environments but might not work the same way in the deployment environment, due to subtle differences between the two environments.
- The system might conform to the system specification but could fail to solve the problem it was supposed to solve for the user, due to flaws in the system design.
Choose the correct statements about system testing and acceptance testing.
- a. Both system testing and acceptance testing typically involve the whole system.
- b. System testing is typically more extensive than acceptance testing.
- c. System testing can include testing for non-functional qualities.
- d. Acceptance testing typically has more user involvement than system testing.
- e. In smaller projects, the developers may do system testing as well, in addition to developer testing.
- f. If system testing is adequately done, we need not do acceptance testing.
(a)(b)(c)(d)(e)(f)
Explanation:
(b) is correct because system testing can aim to cover all specified behaviors and can even go beyond the system specification. Therefore, system testing is typically more extensive than acceptance testing.
(f) is incorrect because it is possible for a system to pass system tests but fail acceptance tests.
Alpha/Beta Testing
Can explain alpha and beta testing
Alpha testing is performed by the users, under controlled conditions set by the software development team.
Beta testing is performed by a selected subset of target users of the system in their natural work setting.
An open beta release is the release of not-yet-production-quality-but-almost-there software to the general population. For example, Google’s Gmail was in 'beta' for many years before the label was finally removed.
[W9.3] Testing: Coverage
Can explain testability
Testability is an indication of how easy it is to test an SUT. As testability depends a lot on the design and implementation. You should try to increase the testability when you design and implement a software. The higher the testability, the easier it is to achieve a better quality software.
Can explain test coverage
Test coverage is a metric used to measure the extent to which testing exercises the code i.e., how much of the code is 'covered' by the tests.
Here are some examples of different coverage criteria:
- Function/method coverage : based on functions executed e.g., testing executed 90 out of 100 functions.
- Statement coverage : based on the number of line of code executed e.g., testing executed 23k out of 25k LOC.
- Decision/branch coverage : based on the decision points exercised e.g., an
if
statement evaluated to bothtrue
andfalse
with separate test cases during testing is considered 'covered'. - Condition coverage : based on the boolean sub-expressions, each evaluated to both true and false with different test cases. Condition coverage is not the same as the decision coverage.
if(x > 2 && x < 44)
is considered one decision point but two conditions.
For 100% branch or decision coverage, two test cases are required:
(x > 2 && x < 44) == true
: [e.g.x == 4
](x > 2 && x < 44) == false
: [e.g.x == 100
]
For 100% condition coverage, three test cases are required
(x > 2) == true
,(x < 44) == true
: [e.g.x == 4
](x < 44) == false
: [e.g.x == 100
](x > 2) == false
: [e.g.x == 0
]
- Path coverage measures coverage in terms of possible paths through a given part of the code executed. 100% path coverage means all possible paths have been executed. A commonly used notation for path analysis is called the Control Flow Graph (CFG).
- Entry/exit coverage measures coverage in terms of possible calls to and exits from the operations in the SUT.
Which of these gives us the highest intensity of testing?
(b)
Explanation: 100% path coverage implies all possible execution paths through the SUT have been tested. This is essentially ‘exhaustive testing’. While this is very hard to achieve for a non-trivial SUT, it technically gives us the highest intensity of testing. If all tests pass at 100% path coverage, the SUT code can be considered ‘bug free’. However, note that path coverage does not include paths that are missing from the code altogether because the programmer left them out by mistake.
Can explain how test coverage works
Measuring coverage is often done using coverage analysis tools. Most IDEs have inbuilt support for measuring test coverage, or at least have plugins that can measure test coverage.
Coverage analysis can be useful in improving the quality of testing e.g., if a set of test cases does not achieve 100% branch coverage, more test cases can be added to cover missed branches.
Measuring code coverage in Intellij IDEA
Can use intermediate features of JUnit
Some intermediate JUnit techniques that may be useful:
- It is possible for a JUnit test case to verify if the SUT throws the right exception.
- JUnit Rules are a way to add additional behavior to a test. e.g. to make a test case use a temporary folder for storing files needed for (or generated by) the test.
- It is possible to write methods thar are automatically run before/after a test method/class. These are useful to do pre/post cleanups for example.
- Testing private methods is possible, although not always necessray
Can explain TDD
Test-Driven Development(TDD)_ advocates writing the tests before writing the SUT, while evolving functionality and tests in small increments. In TDD you first define the precise behavior of the SUT using test cases, and then write the SUT to match the specified behavior. While TDD has its fair share of detractors, there are many who consider it a good way to reduce defects. One big advantage of TDD is that it guarantees the code is testable.
A) In TDD, we write all the test cases before we start writing functional code.
B) Testing tools such as Junit require us to follow TDD.
A) False
Explanation: No, not all. We proceed in small steps, writing tests and functional code in tandem, but writing the test before we write the corresponding functional code.
B) False
Explanation: They can be used for TDD, but they can be used without TDD too.
[W9.4] Test Case Design
Can explain the need for deliberate test case design
Except for trivial
Consider the test cases for adding a string object to a
- Add an item to an empty collection.
- Add an item when there is one item in the collection.
- Add an item when there are 2, 3, .... n items in the collection.
- Add an item that has an English, a French, a Spanish, ... word.
- Add an item that is the same as an existing item.
- Add an item immediately after adding another item.
- Add an item immediately after system startup.
- ...
Exhaustive testing of this operation can take many more test cases.
Program testing can be used to show the presence of bugs, but never to show their absence!
--Edsger Dijkstra
Every test case adds to the cost of testing. In some systems, a single test case can cost thousands of dollars e.g. on-field testing of flight-control software. Therefore, test cases need to be designed to make the best use of testing resources. In particular:
-
Testing should be effective i.e., it finds a high percentage of existing bugs e.g., a set of test cases that finds 60 defects is more effective than a set that finds only 30 defects in the same system.
-
Testing should be efficient i.e., it has a high rate of success (bugs found/test cases) a set of 20 test cases that finds 8 defects is more efficient than another set of 40 test cases that finds the same 8 defects.
For testing to be
Given below is the sample output from a text-based program TriangleDetector
ithat determines whether the three input numbers make up the three sides of a valid triangle. List test cases you would use to test this software. Two sample test cases are given below.
C:\> java TriangleDetector
Enter side 1: 34
Enter side 2: 34
Enter side 3: 32
Can this be a triangle?: Yes
Enter side 1:
Sample test cases,
34,34,34: Yes
0, any valid, any valid: No
In addition to obvious test cases such as
- sum of two sides == third,
- sum of two sides < third ...
We may also devise some interesting test cases such as the ones depicted below.
Note that their applicability depends on the context in which the software is operating.
- Non-integer number, negative numbers,
0
, numbers formatted differently (e.g.13F
), very large numbers (e.g.MAX_INT
), numbers with many decimal places, empty string, ... - Check many triangles one after the other (will the system run out of memory?)
- Backspace, tab, CTRL+C , …
- Introduce a long delay between entering data (will the program be affected by, say the screensaver?), minimize and restore window during the operation, hibernate the system in the middle of a calculation, start with invalid inputs (the system may perform error handling differently for the very first test case), …
- Test on different locale.
The main point to note is how difficult it is to test exhaustively, even on a trivial system.
Explain the why exhaustive testing is not practical using the example of testing newGame()
operation in the Logic
class of a Minesweeper game.
Consider this sequence of test cases:
- Test case 1. Start Minesweeper. Activate
newGame()
and see if it works. - Test case 2. Start Minesweeper. Activate
newGame()
. ActivatenewGame()
again and see if it works. - Test case 3. Start Minesweeper. Activate
newGame()
three times consecutively and see if it works. - …
- Test case 267. Start Minesweeper. Activate
newGame()
267 times consecutively and see if it works.
Well, you get the idea. Exhaustive testing of newGame()
is not practical.
Improving efficiency and effectiveness of test case design can,
- a. improve the quality of the SUT.
- b. save money.
- c. save time spent on test execution.
- d. save effort on writing and maintaining tests.
- e. minimize redundant test cases.
- f. forces us to understand the SUT better.
(a)(b)(c)(d)(e)(f)
Can explain exploratory testing and scripted testing
Here are two alternative approaches to testing a software: Scripted testing and Exploratory testing
-
Scripted testing: First write a set of test cases based on the expected behavior of the SUT, and then perform testing based on that set of test cases.
-
Exploratory testing: Devise test cases on-the-fly, creating new test cases based on the results of the past test cases.
Exploratory testing is ‘the simultaneous learning, test design, and test execution’
Here is an example thought process behind a segment of an exploratory testing session:
“Hmm... looks like feature x is broken. This usually means feature n and k could be broken too; we need to look at them soon. But before that, let us give a good test run to feature y because users can still use the product if feature y works, even if x doesn’t work. Now, if feature y doesn’t work 100%, we have a major problem and this has to be made known to the development team sooner rather than later...”
💡 Exploratory testing is also known as reactive testing, error guessing technique, attack-based testing, and bug hunting.
Exploratory Testing Explained, an online article by James Bach -- James Bach is an industry thought leader in software testing).
Scripted testing requires tests to be written in a scripting language; Manual testing is called exploratory testing.
A) False
Explanation: “Scripted” means test cases are predetermined. They need not be an executable script. However, exploratory testing is usually manual.
Which testing technique is better?
(e)
Explain the concept of exploratory testing using Minesweeper as an example.
When we test the Minesweeper by simply playing it in various ways, especially trying out those that are likely to be buggy, that would be exploratory testing.
Can explain the choice between exploratory testing and scripted testing
Which approach is better – scripted or exploratory? A mix is better.
The success of exploratory testing depends on the tester’s prior experience and intuition. Exploratory testing should be done by experienced testers, using a clear strategy/plan/framework. Ad-hoc exploratory testing by unskilled or inexperienced testers without a clear strategy is not recommended for real-world non-trivial systems. While exploratory testing may allow us to detect some problems in a relatively short time, it is not prudent to use exploratory testing as the sole means of testing a critical system.
Scripted testing is more systematic, and hence, likely to discover more bugs given sufficient time, while exploratory testing would aid in quick error discovery, especially if the tester has a lot of experience in testing similar systems.
In some contexts, you will achieve your testing mission better through a more scripted approach; in other contexts, your mission will benefit more from the ability to create and improve tests as you execute them. I find that most situations benefit from a mix of scripted and exploratory approaches. --
[source: bach-et-explained]
Exploratory Testing Explained, an online article by James Bach -- James Bach is an industry thought leader in software testing).
Scripted testing is better than exploratory testing.
B) False
Explanation: Each has pros and cons. Relying on only one is not recommended. A combination is better.
Can explain positive and negative test cases
A positive test case is when the test is designed to produce an expected/valid behavior. A negative test case is designed to produce a behavior that indicates an invalid/unexpected situation, such as an error message.
Consider testing of the method print(Integer i)
which prints the value of i
.
- A positive test case:
i == new Integer(50)
- A negative test case:
i == null;
Can explain black box and glass box test case design
Test case design can be of three types, based on how much of SUT internal details are considered when designing test cases:
-
Black-box (aka specification-based or responsibility-based) approach: test cases are designed exclusively based on the SUT’s specified external behavior.
-
White-box (aka glass-box or structured or implementation-based) approach: test cases are designed based on what is known about the SUT’s implementation, i.e. the code.
-
Gray-box approach: test case design uses some important information about the implementation. For example, if the implementation of a sort operation uses different algorithms to sort lists shorter than 1000 items and lists longer than 1000 items, more meaningful test cases can then be added to verify the correctness of both algorithms.
Note: these videos are from the Udacity course Software Development Process by Georgia Tech
Can explain test case design for use case based testing
Use cases can be used for system testing and acceptance testing. For example, the main success scenario can be one test case while each variation (due to extensions) can form another test case. However, note that use cases do not specify the exact data entered into the system. Instead, it might say something like user enters his personal data into the system
. Therefore, the tester has to choose data by considering equivalence partitions and boundary values. The combinations of these could result in one use case producing many test cases.
To increase
Every test case adds to the cost of testing. In some systems, a single test case can cost thousands of dollars e.g. on-field testing of flight-control software. Therefore, test cases need to be designed to make the best use of testing resources. In particular:
-
Testing should be effective i.e., it finds a high percentage of existing bugs e.g., a set of test cases that finds 60 defects is more effective than a set that finds only 30 defects in the same system.
-
Testing should be efficient i.e., it has a high rate of success (bugs found/test cases) a set of 20 test cases that finds 8 defects is more efficient than another set of 40 test cases that finds the same 8 defects.
For testing to be
Quality Assurance → Testing → Exploratory and Scripted Testing →
Here are two alternative approaches to testing a software: Scripted testing and Exploratory testing
-
Scripted testing: First write a set of test cases based on the expected behavior of the SUT, and then perform testing based on that set of test cases.
-
Exploratory testing: Devise test cases on-the-fly, creating new test cases based on the results of the past test cases.
Exploratory testing is ‘the simultaneous learning, test design, and test execution’
Here is an example thought process behind a segment of an exploratory testing session:
“Hmm... looks like feature x is broken. This usually means feature n and k could be broken too; we need to look at them soon. But before that, let us give a good test run to feature y because users can still use the product if feature y works, even if x doesn’t work. Now, if feature y doesn’t work 100%, we have a major problem and this has to be made known to the development team sooner rather than later...”
💡 Exploratory testing is also known as reactive testing, error guessing technique, attack-based testing, and bug hunting.
Exploratory Testing Explained, an online article by James Bach -- James Bach is an industry thought leader in software testing).
Scripted testing requires tests to be written in a scripting language; Manual testing is called exploratory testing.
A) False
Explanation: “Scripted” means test cases are predetermined. They need not be an executable script. However, exploratory testing is usually manual.
Which testing technique is better?
(e)
Explain the concept of exploratory testing using Minesweeper as an example.
When we test the Minesweeper by simply playing it in various ways, especially trying out those that are likely to be buggy, that would be exploratory testing.
Equivalence Partitioning
Can explain equivalence partitions
Consider the testing of the following operation.
isValidMonth(m)
: returns true
if m
(and int
) is in the range [1..12]
It is inefficient and impractical to test this method for all integer values [-MIN_INT to MAX_INT]
. Fortunately, there is no need to test all possible input values. For example, if the input value 233
failed to produce the correct result, the input 234
is likely to fail too; there is no need to test both.
In general, most SUTs do not treat each input in a unique way. Instead, they process all possible inputs in a small number of distinct ways. That means a range of inputs is treated the same way inside the SUT. Equivalence partitioning (EP) is a test case design technique that uses the above observation to improve the E&E of testing.
Equivalence partition (aka equivalence class): A group of test inputs that are likely to be processed by the SUT in the same way.
By dividing possible inputs into equivalence partitions we can,
- avoid testing too many inputs from one partition. Testing too many inputs from the same partition is unlikely to find new bugs. This increases the efficiency of testing by reducing redundant test cases.
- ensure all partitions are tested. Missing partitions can result in bugs going unnoticed. This increases the effectiveness of testing by increasing the chance of finding bugs.
Can apply EP for pure functions
Equivalence partitions (EPs) are usually derived from the specifications of the SUT.
These could be EPs for the
- [MIN_INT ... 0] : below the range that produces
true
(producesfalse
) - [1 … 12] : the range that produces
true
- [13 … MAX_INT] : above the range that produces
true
(producesfalse
)
isValidMonth(m)
: returns true
if m
(and int
) is in the range [1..12]
When the SUT has multiple inputs, you should identify EPs for each input.
Consider the method duplicate(String s, int n): String
which returns a String
that contains s
repeated n
times.
Example EPs for s
:
- zero-length strings
- string containing whitespaces
- ...
Example EPs for n
:
0
- negative values
- ...
An EP may not have adjacent values.
Consider the method isPrime(int i): boolean
that returns true if i
is a prime number.
EPs for i
:
- prime numbers
- non-prime numbers
Some inputs have only a small number of possible values and a potentially unique behavior for each value. In those cases we have to consider each value as a partition by itself.
Consider the method showStatusMessage(GameStatus s): String
that returns a unique String
for each of the possible value of s (GameStatus
is an enum
). In this case, each possible value for s
will have to be considered as a partition.
Note that the EP technique is merely a heuristic and not an exact science, especially when applied manually (as opposed to using an automated program analysis tool to derive EPs). The partitions derived depend on how one ‘speculates’ the SUT to behave internally. Applying EP under a glass-box or gray-box approach can yield more precise partitions.
Consider the method EPs given above for the isValidMonth
. A different tester might use these EPs instead:
- [1 … 12] : the range that produces
true
- [all other integers] : the range that produces
false
Some more examples:
Specification | Equivalence partitions |
---|---|
|
[ |
|
[ |
Consider this SUT:
isValidName (String s): boolean
Description: returns true if s
is not null
and not longer than 50 characters.
A. Which one of these is least likely to be an equivalence partition for the parameter s of the isValidName method given below?
B. If you had to choose 3 test cases from the 4 given below, which one will you leave out based on the EP technique?
A. (d)
Explanation: The description does not mention anything about the content of the string. Therefore, the method is unlikely to behave differently for strings consisting of numbers.
B. (a) or (c)
Explanation: both belong to the same EP
Can apply EP for OOP methods
When deciding EPs of OOP methods, we need to identify EPs of all data participants that can potentially influence the behaviour of the method, such as,
- the target object of the method call
- input parameters of the method call
- other data/objects accessed by the method such as global variables. This category may not be applicable if using the black box approach (because the test case designer using the black box approach will not know how the method is implemented)
Consider this method in the DataStack
class:
push(Object o): boolean
- Adds o to the top of the stack if the stack is not full.
- returns
true
if the push operation was a success. - throws
MutabilityException
if the global flagFREEZE==true
.InvalidValueException
if o is null.
EPs:
DataStack
object: [full] [not full]o
: [null] [not null]FREEZE
: [true][false]
Consider a simple Minesweeper app. What are the EPs for the newGame()
method of the Logic
component?
As newGame()
does not have any parameters, the only obvious participant is the Logic
object itself.
Note that if the glass-box or the grey-box approach is used, other associated objects that are involved in the method might also be included as participants. For example, Minefield
object can be considered as another participant of the newGame()
method. Here, the black-box approach is assumed.
Next, let us identify equivalence partitions for each participant. Will the newGame()
method behave differently for different Logic
objects? If yes, how will it differ? In this case, yes, it might behave differently based on the game state. Therefore, the equivalence partitions are:
PRE_GAME
: before the game starts, minefield does not exist yetREADY
: a new minefield has been created and waiting for player’s first moveIN_PLAY
: the current minefield is already in useWON
,LOST
: let us assume thenewGame
behaves the same way for these two values
Consider the Logic
component of the Minesweeper application. What are the EPs for the markCellAt(int x, int y)
method?. The partitions in bold represent valid inputs.
Logic
: PRE_GAME, READY, IN_PLAY, WON, LOSTx
: [MIN_INT..-1] [0..(W-1)] [W..MAX_INT] (we assume a minefield size of WxH)y
: [MIN_INT..-1] [0..(H-1)] [H..MAX_INT]Cell
at(x,y)
: HIDDEN, MARKED, CLEARED
Boundary Value Analysis
Can explain boundary value analysis
Boundary Value Analysis (BVA) is test case design heuristic that is based on the observation that bugs often result from incorrect handling of boundaries of equivalence partitions. This is not surprising, as the end points of the boundary are often used in branching instructions etc. where the programmer can make mistakes.
markCellAt(int x, int y)
operation could contain code such as if (x > 0 && x <= (W-1))
which involves boundaries of x’s equivalence partitions.
BVA suggests that when picking test inputs from an equivalence partition, values near boundaries (i.e. boundary values) are more likely to find bugs.
Boundary values are sometimes called corner cases.
Boundary value analysis recommends testing only values that reside on the equivalence class boundary.
False
Explanation: It does not recommend testing only those values on the boundary. It merely suggests that values on and around a boundary are more likely to cause errors.
Can apply boundary value analysis
Typically, we choose three values around the boundary to test: one value from the boundary, one value just below the boundary, and one value just above the boundary. The number of values to pick depends on other factors, such as the cost of each test case.
Some examples:
Equivalence partition | Some possible boundary values |
---|---|
[1-12] |
0,1,2, 11,12,13 |
[MIN_INT, 0] |
MIN_INT, MIN_INT+1, -1, 0 , 1 |
[any non-null String] |
Empty String, a String of maximum possible length |
[prime numbers] |
No specific boundary |
[non-empty Stack] |
Stack with: one element, two elements, no empty spaces, only one empty space |
Combining Multiple Test Inputs
Can explain the need for strategies to combine test inputs
An SUT can take multiple inputs. You can select values for each input (using equivalence partitioning, boundary value analysis, or some other technique).
an SUT that takes multiple inputs and some values chosen as values for each input:
- Method to test:
calculateGrade(participation, projectGrade, isAbsent, examScore)
- Values to test:
Input valid values to test invalid values to test participation 0, 1, 19, 20 21, 22 projectGrade A, B, C, D, F isAbsent true, false examScore 0, 1, 69, 70, 71, 72
Testing all possible combinations is effective but not efficient. If you test all possible combinations for the above example, you need to test 6x5x2x6=360 cases. Doing so has a higher chance of discovering bugs (i.e. effective) but the number of test cases can be too high (i.e. not efficient). Therefore, we need smarter ways to combine test inputs that are both effective and efficient.
Can explain some basic test input combination strategies
Given below are some basic strategies for generating a set of test cases by combining multiple test input combination strategies.
Let's assume the SUT has the following three inputs and you have selected the given values for testing:
SUT: foo(p1 char, p2 int, p3 boolean)
Values to test:
Input | Values |
---|---|
p1 | a, b, c |
p2 | 1, 2, 3 |
p3 | T, F |
The all combinations strategy generates test cases for each unique combination of test inputs.
the strategy generates 3x3x2=18 test cases
Test Case | p1 | p2 | p3 |
---|---|---|---|
1 | a | 1 | T |
2 | a | 1 | F |
3 | a | 2 | T |
... | ... | ... | ... |
18 | c | 3 | F |
The at least once strategy includes each test input at least once.
this strategy generates 3 test cases.
Test Case | p1 | p2 | p3 |
---|---|---|---|
1 | a | 1 | T |
2 | b | 2 | F |
3 | c | 3 | VV/IV |
VV/IV = Any Valid Value / Any Invalid Value
The all pairs strategy creates test cases so that for any given pair of inputs, all combinations between them are tested. It is based on the observations that a bug is rarely the result of more than two interacting factors. The resulting number of test cases is lower than the all combinations strategy, but higher than the at least once approach.
this strategy generates 9 test cases:
Let's first consider inputs p1 and p2:
Input | Values |
---|---|
p1 | a, b, c |
p2 | 1, 2, 3 |
These values can generate
Next, let's consider p1 and p3.
Input | Values |
---|---|
p1 | a, b, c |
p3 | T, F |
These values can generate
Similarly, inputs p2 and p3 generates another 6 combinations.
The 9 test cases given below covers all those 9+6+6 combinations.
Test Case | p1 | p2 | p3 |
---|---|---|---|
1 | a | 1 | T |
2 | a | 2 | T |
3 | a | 3 | F |
4 | b | 1 | F |
5 | b | 2 | T |
6 | b | 3 | F |
7 | c | 1 | T |
8 | c | 2 | F |
9 | c | 3 | T |
A variation of this strategy is to test all pairs of inputs but only for inputs that could influence each other.
Testing all pairs between p1 and p3 only while ensuring all p3 values are tested at least once
Test Case | p1 | p2 | p3 |
---|---|---|---|
1 | a | 1 | T |
2 | a | 2 | F |
3 | b | 3 | T |
4 | b | VV/IV | F |
5 | c | VV/IV | T |
6 | c | VV/IV | F |
The random strategy generates test cases using one of the other strategies and then pick a subset randomly (presumably because the original set of test cases is too big).
There are other strategies that can be used too.
Can apply heuristic ‘each valid input at least once in a positive test case’
Consider the following scenario.
SUT: printLabel(fruitName String, unitPrice int)
Selected values for fruitName
(invalid values are underlined ):
Values | Explanation |
---|---|
Apple | Label format is round |
Banana | Label format is oval |
Cherry | Label format is square |
Dog | Not a valid fruit |
Selected values for unitPrice
:
Values | Explanation |
---|---|
1 | Only one digit |
20 | Two digits |
0 | Invalid because 0 is not a valid price |
-1 | Invalid because negative prices are not allowed |
Suppose these are the test cases being considered.
Case | fruitName | unitPrice | Expected |
---|---|---|---|
1 | Apple | 1 | Print label |
2 | Banana | 20 | Print label |
3 | Cherry | 0 | Error message “invalid price” |
4 | Dog | -1 | Error message “invalid fruit" |
It looks like the test cases were created using the at least once strategy. After running these tests can we confirm that square-format label printing is done correctly?
- Answer: No.
- Reason:
Cherry
-- the only input that can produce a square-format label -- is in a negative test case which produces an error message instead of a label. If there is a bug in the code that prints labels in square-format, these tests cases will not trigger that bug.
In this case a useful heuristic to apply is each valid input must appear at least once in a positive test case. Cherry
is a valid test input and we must ensure that it appears at least once in a positive test case. Here are the updated test cases after applying that heuristic.
Case | fruitName | unitPrice | Expected |
---|---|---|---|
1 | Apple | 1 | Print round label |
2 | Banana | 20 | Print oval label |
2.1 | Cherry | VV | Print square label |
3 | VV | 0 | Error message “invalid price” |
4 | Dog | -1 | Error message “invalid fruit" |
VV/IV = Any Invalid or Valid Value VV=Any Valid Value
Can apply heuristic ‘no more than one invalid input in a test case’
Consider the
Case | fruitName | unitPrice | Expected |
---|---|---|---|
1 | Apple | 1 | Print round label |
2 | Banana | 20 | Print oval label |
2.1 | Cherry | VV | Print square label |
3 | VV | 0 | Error message “invalid price” |
4 | Dog | -1 | Error message “invalid fruit" |
VV/IV = Any Invalid or Valid Value VV=Any Valid Value
After running these test cases can you be sure that the error message “invalid price” is shown for negative prices?
- Answer: No.
- Reason:
-1
-- the only input that is a negative price -– is in a test case that produces the error message “invalid fruit”.
In this case a useful heuristic to apply is no more than one invalid input in a test case. After applying that, we get the following test cases.
Case | fruitName | unitPrice | Expected |
---|---|---|---|
1 | Apple | 1 | Print round label |
2 | Banana | 20 | Print oval label |
2.1 | Cherry | VV | Print square label |
3 | VV | 0 | Error message “invalid price” |
4 | VV | -1 | Error message “invalid price" |
4.1 | Dog | VV | Error message “invalid fruit" |
VV/IV = Any Invalid or Valid Value VV=Any Valid Value
Applying the heuristics covered so far, we can determine the precise number of test cases required to test any given SUT effectively.
False
Explanation: These heuristics are, well, heuristics only. They will help you to make better decisions about test case design. However, they are speculative in nature (especially, when testing in black-box fashion) and cannot give you precise number of test cases.
Can apply multiple test input combination techniques together
Consider the calculateGrade scenario given below:
- SUT :
calculateGrade(participation, projectGrade, isAbsent, examScore)
- Values to test: invalid values are underlined
- participation: 0, 1, 19, 20, 21, 22
- projectGrade: A, B, C, D, F
- isAbsent: true, false
- examScore: 0, 1, 69, 70, 71, 72
To get the first cut of test cases, let’s apply the at least once strategy.
Test cases for calculateGrade V1
Case No. | participation | projectGrade | isAbsent | examScore | Expected |
---|---|---|---|---|---|
1 | 0 | A | true | 0 | ... |
2 | 1 | B | false | 1 | ... |
3 | 19 | C | VV/IV | 69 | ... |
4 | 20 | D | VV/IV | 70 | ... |
5 | 21 | F | VV/IV | 71 | Err Msg |
6 | 22 | VV/IV | VV/IV | 72 | Err Msg |
VV/IV = Any Valid or Invalid Value, Err Msg = Error Message
Next, let’s apply the each valid input at least once in a positive test case heuristic. Test case 5 has a valid value for projectGrade=F
that doesn't appear in any other positive test case. Let's replace test case 5 with 5.1 and 5.2 to rectify that.
Test cases for calculateGrade V2
Case No. | participation | projectGrade | isAbsent | examScore | Expected |
---|---|---|---|---|---|
1 | 0 | A | true | 0 | ... |
2 | 1 | B | false | 1 | ... |
3 | 19 | C | VV | 69 | ... |
4 | 20 | D | VV | 70 | ... |
5.1 | VV | F | VV | VV | ... |
5.2 | 21 | VV/IV | VV/IV | 71 | Err Msg |
6 | 22 | VV/IV | VV/IV | 72 | Err Msg |
VV = Any Valid Value VV/IV = Any Valid or Invalid Value
Next, we apply the no more than one invalid input in a test case heuristic. Test cases 5.2 and 6 don't follow that heuristic. Let's rectify the situation as follows:
Test cases for calculateGrade V3
Case No. | participation | projectGrade | isAbsent | examScore | Expected |
---|---|---|---|---|---|
1 | 0 | A | true | 0 | ... |
2 | 1 | B | false | 1 | ... |
3 | 19 | C | VV | 69 | ... |
4 | 20 | D | VV | 70 | ... |
5.1 | VV | F | VV | VV | ... |
5.2 | 21 | VV | VV | VV | Err Msg |
5.3 | 22 | VV | VV | VV | Err Msg |
6.1 | VV | VV | VV | 71 | Err Msg |
6.2 | VV | VV | VV | 72 | Err Msg |
Next, let us assume that there is a dependency between the inputs examScore
and isAbsent
such that an absent student can only have examScore=0
. To cater for the hidden invalid case arising from this, we can add a new test case where isAbsent=true
and examScore!=0
. In addition, test cases 3-6.2 should have isAbsent=false
so that the input remains valid.
Test cases for calculateGrade V4
Case No. | participation | projectGrade | isAbsent | examScore | Expected |
---|---|---|---|---|---|
1 | 0 | A | true | 0 | ... |
2 | 1 | B | false | 1 | ... |
3 | 19 | C | false | 69 | ... |
4 | 20 | D | false | 70 | ... |
5.1 | VV | F | false | VV | ... |
5.2 | 21 | VV | false | VV | Err Msg |
5.3 | 22 | VV | false | VV | Err Msg |
6.1 | VV | VV | false | 71 | Err Msg |
6.2 | VV | VV | false | 72 | Err Msg |
7 | VV | VV | true | !=0 | Err Msg |
Which of these contradict the heuristics recommended when creating test cases with multiple inputs?
(a) inputs.
Explanation: If you test all invalid test inputs together, you will not know if each one of the invalid inputs are handled correctly by the SUT. This is because most SUTs return an error message upon encountering the first invalid input.
Apply heuristics for combining multiple test inputs to improve the E&E of the following test cases, assuming all 6 values in the table need to be tested. underlines indicate invalid values. Point out where the heuristics are contradicted and how to improve the test cases.
SUT: consume(food, drink)
Test case | food | drink |
---|---|---|
TC1 | bread | water |
TC2 | rice | lava |
TC3 | rock | acid |
[W9.5] Other QA Techniques
Can explain software quality assurance
Software Quality Assurance (QA) is the process of ensuring that the software being built has the required levels of quality.
While testing is the most common activity used in QA, there are other complementary techniques such as static analysis, code reviews, and formal verification.
Can explain validation and verification
Quality Assurance = Validation + Verification
QA involves checking two aspects:
- Validation: are we building the right system i.e., are the requirements correct?
- Verification: are we building the system right i.e., are the requirements implemented correctly?
Whether something belongs under validation or verification is not that important. What is more important is both are done, instead of limiting to verification (i.e., remember that the requirements can be wrong too).
Choose the correct statements about validation and verification.
- a. Validation: Are we building the right product?, Verification: Are we building the product right?
- b. It is very important to clearly distinguish between validation and verification.
- c. The important thing about validation and verification is to remember to pay adequate attention to both.
- d. Developer-testing is more about verification than validation.
- e. QA covers both validation and verification.
- f. A system crash is more likely to be a verification failure than a validation failure.
(a)(b)(c)(d)(e)(f)
Explanation:
Whether something belongs under validation or verification is not that important. What is more important is that we do both.
Developer testing is more about bugs in code, rather than bugs in the requirements.
In QA, system testing is more about verification (does the system follow the specification?) and acceptance testings is more about validation (does the system solve the user’s problem?).
A system crash is more likely to be a bug in the code, not in the requirements.
Can explain code reviews
Code review is the systematic examination code with the intention of finding where the code can be improved.
Reviews can be done in various forms. Some examples below:
-
In
pair programming - As pair programming involves two programmers working on the same code at the same time, there is an implicit review of the code by the other member of the pair.
Pair Programming:
Pair programming is an agile software development technique in which two programmers work together at one workstation. One, the driver, writes code while the other, the observer or navigator, reviews each line of code as it is typed in. The two programmers switch roles frequently. [source: Wikipedia]
A good introduction to pair programming:
-
Pull Request reviews
- Project Management Platforms such as GitHub and BitBucket allows the new code to be proposed as Pull Requests and provides the ability for others to review the code in the PR.
-
Formal inspections
-
Inspections involve a group of people systematically examining a project artifacts to discover defects. Members of the inspection team play various roles during the process, such as:
- the author - the creator of the artifact
- the moderator - the planner and executor of the inspection meeting
- the secretary - the recorder of the findings of the inspection
- the inspector/reviewer - the one who inspects/reviews the artifact.
-
Advantages of code reviews over testing:
- It can detect functionality defects as well as other problems such as coding standard violations.
- Can verify non-code artifacts and incomplete code
- Do not require test drivers or stubs.
Disadvantages:
- It is a manual process and therefore, error prone.
Can explain static analysis
Static analysis: Static analysis is the analysis of code without actually executing the code.
Static analysis of code can find useful information such unused variables, unhandled exceptions, style errors, and statistics. Most modern IDEs come with some inbuilt static analysis capabilities. For example, an IDE can highlight unused variables as you type the code into the editor.
Higher-end static analyzer tools can perform for more complex analysis such as locating potential bugs, memory leaks, inefficient code structures etc.
Some example static analyzer for Java:
Linters are a subset of static analyzers that specifically aim to locate areas where the code can be made 'cleaner'.
Can explain formal verification
Formal verification uses mathematical techniques to prove the correctness of a program.
by Eric Hehner
Advantages:
- Formal verification can be used to prove the absence of errors. In contrast, testing can only prove the presence of error, not their absence.
Disadvantages:
- It only proves the compliance with the specification, but not the actual utility of the software.
- It requires highly specialized notations and knowledge which makes it an expensive technique to administer. Therefore, formal verifications are more commonly used in safety-critical software such as flight control systems.
Testing cannot prove the absence of errors. It can only prove the presence of errors. However, formal methods can prove the absence of errors.
True
Explanation: While using formal methods is more expensive than testing, it indeed can prove the correctness of a piece of software conclusively, in certain contexts. Getting such proof via testing requires exhaustive testing, which is not practical to do in most cases.
Project Milestone: v1.2
Move code towards v2.0 in small steps, start documenting design/implementation details in DG.
v1.2 Summary of Milestone
Milestone | Minimum acceptable performance to consider as 'reached' |
---|---|
Contributed code to the product as described in mid-v1.2 progress guide | some code merged |
Described implementation details in the Developer Guide | some text and some diagrams added to the developer guide (at least in a PR), comprising at least one page worth of content |
Issue tracker set up | As explained in |
v1.2 managed using GitHub features (issue tracker, milestones, etc.) | Milestone v1.2 managed as explained in |
Issue tracker setup
We recommend you configure the issue tracker of the main
repo as follows:
- Delete existing labels and add the following labels.
💡 Issue type labels are useful from the beginning of the project. The other labels are needed only when you start implementing the features.
Issue type labels:
type.Epic
: A big feature which can be broken down into smaller stories e.g. searchtype.Story
: A user storytype.Enhancement
: An enhancement to an existing storytype.Task
: Something that needs to be done, but not a story, bug, or an epic. e.g. Move testing code into a new folder)type.Bug
: A bug
Status labels:
status.Ongoing
: The issue is currently being worked on. note: remove this label before closing an issue.
Priority labels:
priority.High
: Must dopriority.Medium
: Nice to havepriority.Low
: Unlikely to do
Bug Severity labels:
severity.Low
: A flaw that is unlikely to affect normal operations of the product. Appears only in very rare situations and causes a minor inconvenience only.severity.Medium
: A flaw that causes occasional inconvenience to some users but they can continue to use the product.severity.High
: A flaw that affects most users and causes major problems for users. i.e., makes the product almost unusable for most users.
-
Create following milestones :
v1.0
,v1.1
,v1.2
,v1.3
,v1.4
, -
You may configure other project settings as you wish. e.g. more labels, more milestones
Project Schedule Tracking
In general, use the issue tracker (Milestones, Issues, PRs, Tags, Releases, and Labels) for assigning, scheduling, and tracking all noteworthy project tasks, including user stories. Update the issue tracker regularly to reflect the current status of the project. You can also use GitHub's Projects feature to manage the project, but keep it linked to the issue tracker as much as you can.
Using Issues:
During the initial stages (latest by the start of v1.2):
-
Record each of the user stories you plan to deliver as an issue in the issue tracker. e.g.
Title: As a user I can add a deadline
Description: ... so that I can keep track of my deadlines
-
Assign the
type.*
andpriority.*
labels to those issues. -
Formalize the project plan by assigning relevant issues to the corresponding milestone.
From milestone v1.2:
-
Define project tasks as issues. When you start implementing a user story (or a feature), break it down to smaller tasks if necessary. Define reasonable sized, standalone tasks. Create issues for each of those tasks so that they can be tracked.e.g.
-
A typical task should be able to done by one person, in a few hours.
- Bad (reasons: not a one-person task, not small enough):
Write the Developer Guide
- Good:
Update class diagram in the Developer Guide for v1.4
- Bad (reasons: not a one-person task, not small enough):
-
There is no need to break things into VERY small tasks. Keep them as big as possible, but they should be no bigger than what you are going to assign a single person to do within a week. eg.,
- Bad:
Implementing parser
(reason: too big). - Good:
Implementing parser support for adding of floating tasks
- Bad:
-
Do not track things taken for granted. e.g.,
push code to repo
should not be a task to track. In the example given under the previous point, it is taken for granted that the owner will also (a) test the code and (b) push to the repo when it is ready. Those two need not be tracked as separate tasks. -
Write a descriptive title for the issue. e.g.
Add support for the 'undo' command to the parser
- Omit redundant details. In some cases, the issue title is enough to describe the task. In that case, no need to repeat it in the issue description. There is no need for well-crafted and detailed descriptions for tasks. A minimal description is enough. Similarly, labels such as
priority
can be omitted if you think they don't help you.
- Omit redundant details. In some cases, the issue title is enough to describe the task. In that case, no need to repeat it in the issue description. There is no need for well-crafted and detailed descriptions for tasks. A minimal description is enough. Similarly, labels such as
-
-
Assign tasks (i.e., issues) to the corresponding team members using the
assignees
field. Normally, there should be some ongoing tasks and some pending tasks against each team member at any point. -
Optionally, you can use
status.ongoing
label to indicate issues currently ongoing.
Using Milestones:
We recommend you do proper milestone management starting from v1.2. Given below are the conditions to satisfy for a milestone to be considered properly managed:
Planning a Milestone:
-
Issues assigned to the milestone, team members assigned to issues: Used GitHub milestones to indicate which issues are to be handled for which milestone by assigning issues to suitable milestones. Also make sure those issues are assigned to team members. Note that you can change the milestone plan along the way as necessary.
-
Deadline set for the milestones (in the GitHub milestone). Your internal milestones can be set earlier than the deadlines we have set, to give you a buffer.
Wrapping up a Milestone:
-
A working product tagged with the correct tag (e.g.
v1.2
) and is pushed to the main repo
or a product release done on GitHub. A product release is optional for v1.2 but required from from v1.3. Click here to see an example release. -
All tests passing on Travis for the version tagged/released.
-
Milestone updated to match the product i.e. all issues completed and PRs merged for the milestone should be assigned to the milestone. Incomplete issues/PRs should be moved to a future milestone.
-
Milestone closed.
-
If necessary, future milestones are revised based on what you experienced in the current milestone e.g. if you could not finish all issues assigned to the current milestone, it is a sign that you overestimated how much you can do in a week, which means you might want to reduce the issues assigned to future milestones to match that observation.
v1.2 Project Management
- Manage the milestone v1.2 as explained in
[Admin Appendix E: GitHub: Project Schedule Tracking] .
v1.2 Product
- Merge some code into the
master
branch of your team repo. This is expected to be a user-visible enhancement.
v1.2 Documentation
-
User Guide: Update as necessary.
- If a feature has been released in this version, remove the
Coming in v2.0
annotation from that feature. Also replace UI mock-ups with actual screenshots. - If a feature design has changed, update the descriptions accordingly.
- If a feature has been released in this version, remove the
-
Developer Guide:
- Each member should describe the implementation of at least one enhancement she has added (or planning to add).
Expected length: 1+ page per person in v1.2 and 3 pages per person in v1.3/v1.4 - The description can contain things such as,
- How the feature is implemented.
- Why it is implemented that way.
- Alternatives considered.
- The stated objective is to explain the implementation to a future developer, but a hidden objective is to show evidence that you can document deeply-technical content using prose, examples, diagrams, code snippets, etc. appropriately. To that end, you may also describe features that you plan to implement in the future, even beyond v1.4 (hypothetically).
- For an example, see the description of the undo/redo feature implementation in the AddressBook-Level4 developer guide.
- Each member should describe the implementation of at least one enhancement she has added (or planning to add).
v1.2 Demo
Do an informal demo of the new feature during the tutorial. To save time, we recommend that one member demos all new features, using the commit tagged as v1.2
in the master
branch i.e. only features included in the current release should be demoed.
Questions to discuss during tutorial:
Divide these questions among team members. Be prepared to answer questions allocated to you.
Q1
- Explain what Gradle is. How is it used in AB4?
- Explain what Travis is. How is it used in AB4?
- Which integration approach is used by the project?
- What is test coverage? How is it used in AB4?
- How to measure coverage in Intellij?
- How do you ensure some clean up code is run after each JUnit test case?
Q2
- What is unit/integration/system/acceptance testing?
- How is each one done in AB4?
- What’s the difference between unit testing and integration testing?
- What’s the difference between system testing and acceptance testing?
Q3
- What’s the difference between validation and verification?
Acceptance tests are validation tests or verification tests? - Give an example of static analysis being used in Intellij
Q4
- Explain and justify: testing should be efficient and effective
- Explain how exploratory and scripted testing is used in AB4/project
- Give an example of a negative test case in AB4/project
- Explain grey-box test case design
Q5
- Explain: Equivalence Partition improve E&E of testing
- What are the EPs for the parameter
day
of this method/** * Returns true if the three values represent a valid day */ boolean isValidDay(int year, int month, int day){ }
Q6
- Explain: Boundary Value Analysis improves E&E of testing
- What are the boundary values for the parameter
day
in the question above? - How can EP and BVA heuristics be used in AB4/project? Hint: See [AB4 Learning Outcomes: LO-TestCaseDesignHeuristics]
Q7
See the chapter on Code Quality.
Suggest ways to improve the quality of the code below.
import java.io.FileNotFoundException;
import java.util.*;
public class CliApp {
//...
private static final String MESSAGE_COMMAND_HELP_PARAMETERS = "Parameters: %1$s";
private static final String MESSAGE_COMMAND_HELP_EXAMPLE = "Example: %1$s";
private static final String MESSAGE_DISPLAY_PERSON_DATA = "%1$s Phone Number: %2$s Email: %3$s";
private static final String GOODBYE_MESSAGE = "Exiting Address Book... Good bye!";
private static final String MESSAGE_INVALID_COMMAND_FORMAT = "Invalid command format: %1$s";
//...
/**
* List of all persons in the address book.
*/
private static final ArrayList<String> person = new ArrayList<>();
//...
public static void main(String[] args) {
String userCommand = "nothing"; boolean exit = false;
if (args.length > 0) {
showWelcomeMessage();
processProgramArgs(args);
loadDataFromStorage();
while (!exit) {
System.out.print("Enter command: ");
userCommand = SCANNER.nextLine();
userCommand = userCommand.trim();
showToUser(userCommand);
String feedback = executeCommand(userCommand);
showResultToUser(feedback);
}
} else {
showToUser("Incorrect usage!");
}
}
private static void showResultToUser(String feedback) {
switch (feedback) {
case "0":
feedback = "Good (0)";
break;
case "1":
feedback = "Better (1)";
break;
}
try {
writeToFile(feedback);
} catch (FileNotFoundException e) {
//e.printStackTrace();
}
}
private static void matcher(String s1, String s2) {
// ...
}
/**
* Show the {@code message} to the user
*/
private static void showToUser(String message) {
System.out.println(LINE_PREFIX + message.trim()); // add LINE_PREFIX in front
}
// ...
}
Q8
Apply heuristics for combining multiple test inputs to improve the E&E of the following test cases, assuming all 6 values in the table need to be tested. underlines indicate invalid values. Point out where the heuristics are contradicted and how to improve the test cases.
SUT: consume(food, drink)
Test case | food | drink |
---|---|---|
TC1 | bread | water |
TC2 | rice | lava |
TC3 | rock | acid |
Move code towards v2.0 in small steps, start documenting design/implementation details in DG.
v1.2 Summary of Milestone
Milestone | Minimum acceptable performance to consider as 'reached' |
---|---|
Contributed code to the product as described in mid-v1.2 progress guide | some code merged |
Described implementation details in the Developer Guide | some text and some diagrams added to the developer guide (at least in a PR), comprising at least one page worth of content |
Issue tracker set up | As explained in |
v1.2 managed using GitHub features (issue tracker, milestones, etc.) | Milestone v1.2 managed as explained in |
Issue tracker setup
We recommend you configure the issue tracker of the main
repo as follows:
- Delete existing labels and add the following labels.
💡 Issue type labels are useful from the beginning of the project. The other labels are needed only when you start implementing the features.
Issue type labels:
type.Epic
: A big feature which can be broken down into smaller stories e.g. searchtype.Story
: A user storytype.Enhancement
: An enhancement to an existing storytype.Task
: Something that needs to be done, but not a story, bug, or an epic. e.g. Move testing code into a new folder)type.Bug
: A bug
Status labels:
status.Ongoing
: The issue is currently being worked on. note: remove this label before closing an issue.
Priority labels:
priority.High
: Must dopriority.Medium
: Nice to havepriority.Low
: Unlikely to do
Bug Severity labels:
severity.Low
: A flaw that is unlikely to affect normal operations of the product. Appears only in very rare situations and causes a minor inconvenience only.severity.Medium
: A flaw that causes occasional inconvenience to some users but they can continue to use the product.severity.High
: A flaw that affects most users and causes major problems for users. i.e., makes the product almost unusable for most users.
-
Create following milestones :
v1.0
,v1.1
,v1.2
,v1.3
,v1.4
, -
You may configure other project settings as you wish. e.g. more labels, more milestones
Project Schedule Tracking
In general, use the issue tracker (Milestones, Issues, PRs, Tags, Releases, and Labels) for assigning, scheduling, and tracking all noteworthy project tasks, including user stories. Update the issue tracker regularly to reflect the current status of the project. You can also use GitHub's Projects feature to manage the project, but keep it linked to the issue tracker as much as you can.
Using Issues:
During the initial stages (latest by the start of v1.2):
-
Record each of the user stories you plan to deliver as an issue in the issue tracker. e.g.
Title: As a user I can add a deadline
Description: ... so that I can keep track of my deadlines
-
Assign the
type.*
andpriority.*
labels to those issues. -
Formalize the project plan by assigning relevant issues to the corresponding milestone.
From milestone v1.2:
-
Define project tasks as issues. When you start implementing a user story (or a feature), break it down to smaller tasks if necessary. Define reasonable sized, standalone tasks. Create issues for each of those tasks so that they can be tracked.e.g.
-
A typical task should be able to done by one person, in a few hours.
- Bad (reasons: not a one-person task, not small enough):
Write the Developer Guide
- Good:
Update class diagram in the Developer Guide for v1.4
- Bad (reasons: not a one-person task, not small enough):
-
There is no need to break things into VERY small tasks. Keep them as big as possible, but they should be no bigger than what you are going to assign a single person to do within a week. eg.,
- Bad:
Implementing parser
(reason: too big). - Good:
Implementing parser support for adding of floating tasks
- Bad:
-
Do not track things taken for granted. e.g.,
push code to repo
should not be a task to track. In the example given under the previous point, it is taken for granted that the owner will also (a) test the code and (b) push to the repo when it is ready. Those two need not be tracked as separate tasks. -
Write a descriptive title for the issue. e.g.
Add support for the 'undo' command to the parser
- Omit redundant details. In some cases, the issue title is enough to describe the task. In that case, no need to repeat it in the issue description. There is no need for well-crafted and detailed descriptions for tasks. A minimal description is enough. Similarly, labels such as
priority
can be omitted if you think they don't help you.
- Omit redundant details. In some cases, the issue title is enough to describe the task. In that case, no need to repeat it in the issue description. There is no need for well-crafted and detailed descriptions for tasks. A minimal description is enough. Similarly, labels such as
-
-
Assign tasks (i.e., issues) to the corresponding team members using the
assignees
field. Normally, there should be some ongoing tasks and some pending tasks against each team member at any point. -
Optionally, you can use
status.ongoing
label to indicate issues currently ongoing.
Using Milestones:
We recommend you do proper milestone management starting from v1.2. Given below are the conditions to satisfy for a milestone to be considered properly managed:
Planning a Milestone:
-
Issues assigned to the milestone, team members assigned to issues: Used GitHub milestones to indicate which issues are to be handled for which milestone by assigning issues to suitable milestones. Also make sure those issues are assigned to team members. Note that you can change the milestone plan along the way as necessary.
-
Deadline set for the milestones (in the GitHub milestone). Your internal milestones can be set earlier than the deadlines we have set, to give you a buffer.
Wrapping up a Milestone:
-
A working product tagged with the correct tag (e.g.
v1.2
) and is pushed to the main repo
or a product release done on GitHub. A product release is optional for v1.2 but required from from v1.3. Click here to see an example release. -
All tests passing on Travis for the version tagged/released.
-
Milestone updated to match the product i.e. all issues completed and PRs merged for the milestone should be assigned to the milestone. Incomplete issues/PRs should be moved to a future milestone.
-
Milestone closed.
-
If necessary, future milestones are revised based on what you experienced in the current milestone e.g. if you could not finish all issues assigned to the current milestone, it is a sign that you overestimated how much you can do in a week, which means you might want to reduce the issues assigned to future milestones to match that observation.
v1.2 Project Management
- Manage the milestone v1.2 as explained in
[Admin Appendix E: GitHub: Project Schedule Tracking] .
v1.2 Product
- Merge some code into the
master
branch of your team repo. This is expected to be a user-visible enhancement.
v1.2 Documentation
-
User Guide: Update as necessary.
- If a feature has been released in this version, remove the
Coming in v2.0
annotation from that feature. Also replace UI mock-ups with actual screenshots. - If a feature design has changed, update the descriptions accordingly.
- If a feature has been released in this version, remove the
-
Developer Guide:
- Each member should describe the implementation of at least one enhancement she has added (or planning to add).
Expected length: 1+ page per person in v1.2 and 3 pages per person in v1.3/v1.4 - The description can contain things such as,
- How the feature is implemented.
- Why it is implemented that way.
- Alternatives considered.
- The stated objective is to explain the implementation to a future developer, but a hidden objective is to show evidence that you can document deeply-technical content using prose, examples, diagrams, code snippets, etc. appropriately. To that end, you may also describe features that you plan to implement in the future, even beyond v1.4 (hypothetically).
- For an example, see the description of the undo/redo feature implementation in the AddressBook-Level4 developer guide.
- Each member should describe the implementation of at least one enhancement she has added (or planning to add).
v1.2 Demo
Do an informal demo of the new feature during the tutorial. To save time, we recommend that one member demos all new features, using the commit tagged as v1.2
in the master
branch i.e. only features included in the current release should be demoed.